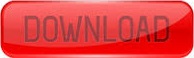

What I hear instead is an incessant desire by business analysts for more data sources, whether these be public data off the web or other enterprise data. I hardly ever hear enterprises complaining about the operational cost of their data warehouse. In this use case, the pain of assembling composite data is justified by the better decision making that results. In spite of these issues, data warehouses have been a huge success for customer facing data, and are in use by most major enterprises. It is expensive to figure out ground truth in such situations. They might be in a food court, one might have replaced the other in a stand-alone location or this might be a data error.
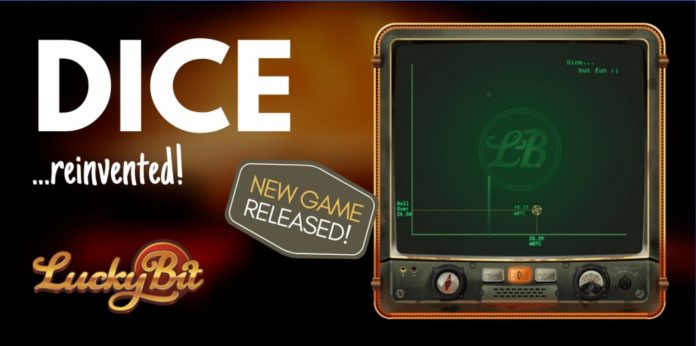
Equally challenging is two restaurants at the same address. Stonebraker are the same entity or different ones. One has to decide if Mike Stonebraker and M.R. The second is that deduplication is hard. This results from using nicknames for people or products, having stale addresses for suppliers, having incorrect ages for people, etc. A rule of thumb is that 10% of your data is incorrect. The problem was that planners underestimated the difficulty of the data integration challenge. The typical data warehouse project in the 1990’s was a factor of two over budget and a factor of two late.
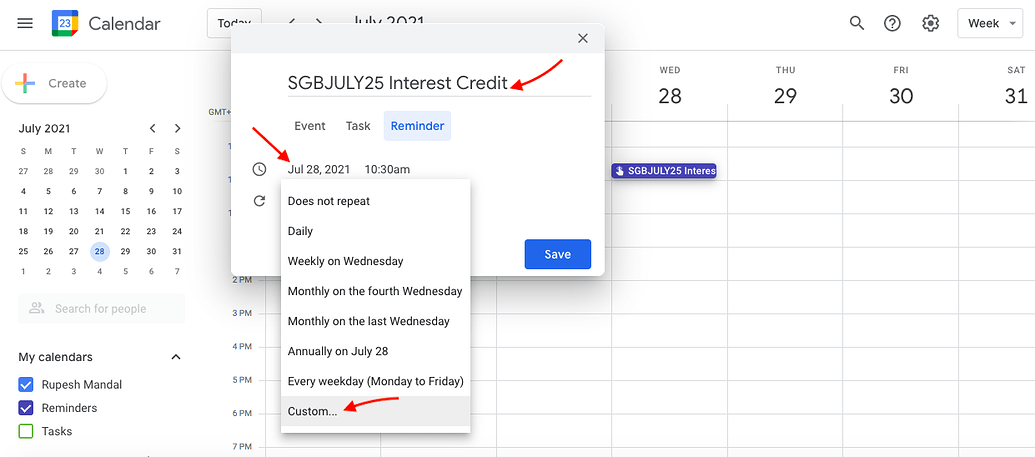
A skilled programmer is required to perform most of the steps in the methodology for each data source.ĭata integration and cleaning is fundamentally difficult. This limits the plausible scope of data warehouses. Hence, an upfront global schema is incredibly difficult to construct for a broad domain. At the end of this time, their result was two years out of date, and was declared a failure. A team was charged with doing this and would work on it for a couple of years. About this time, there was a push in many enterprises to write an enterprise-wide schema for all company objects. It became apparent that this methodology scales to perhaps a dozen data sources, because of three big issues:Ī global schema is needed up front. Hopefully, this will become less problematic in the future with more open source and standardized formats.Ĭ) Have him write transformations, often in a scripting language, and any necessary cleaning routinesĭ) Have him write a script to load the data into a data warehouse Historically, writing such “connectors” was a lot of work because of arcane formats.
#Query golden records how to
The basic methodology was:ī) Send a programmer out to the owner of each data source and have him figure out how to do the extraction.
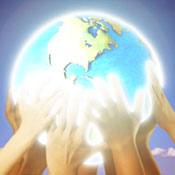
In the late 1990s and early 2000’s there was a giant “pile on” as essentially all enterprises followed the lead of retailers and organized their customer-facing data into a data warehouse.Ī new industry was spawned to support the loading of data warehouses, called extract, transform, and load (ETL) systems. A typical retail data warehouse paid for itself within a year through better buying and stock rotation decisions. In other words, a buyer would figure out that pet rocks are “out” and barbie dolls are “in.” Hence, he would tie up the barbie doll factory with a big order and move the pet rocks up front and discount them to get rid of them. This data warehouse kept historical sales data for a couple of years and was used by the buyers in the organization to rotate stock. To do this they needed to extract data from in-store sales systems, transform it into a predefined common representation (think of this as a global schema), and then load it into a data warehouse. In my opinion, the topic began with the major retailers in the 1990s consolidating their sales data into a data warehouse. I will start this treatise with a history of two major themes in data integration.
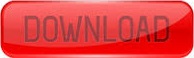